“To effectively contend with questions of fairness, the machine learning community cannot reduce fairness to a technical question. Instead, it must increasingly and explicitly adopt an agenda of broad institutional change and a stance on the political impacts of the technology itself.”
Does this statement seem obvious to you? It seemed obvious to me when I first read it – it totally fits in with the FAT*-style narrative that artificial intelligence simply amplifies the systemic biases of society and the STS-style argument that, ahem, artifacts do in fact have politics.
But, oh boy, this statement was the center of quite a bit of controversy at this past year’s International Conference on Machine Learning (ICML). In fact, this statement was the center of a hosted debate during the conference. There were two sides to this debate: no, we cannot reduce fairness to a technical question, and yes – we can and should reduce fairness to a technical question!
And while you might (maybe strongly) disagree with one side or another, it’s important to note that this debate got a few hundred machine learning researchers and practitioners in a room to contend with a big ethical dilemma.
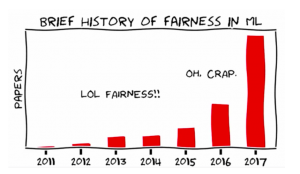
But what you might also note is that this kind of ethics discussion – one that centers around causal inference, statistical measures, impossibility theorems – feels very different from the design-oriented discussions we’ve been having in class. Furthermore, if you go talk to actual moral philosophers interested in AI (as I have had to do for my thesis), you’ll find that the discussion differs even more – and you’ll find yourself wondering if moral reasoning is useful, and if so, how useful (and can you prove it?).
My point here is this: lots of people are worried about the ethical dilemmas of AI (see my ecosystem map below), but we (those of us in different academic fields, industries, citizens, the government, news media) all seem to be speaking very different languages when it comes to identifying problems and solutions. And while some fields are making great efforts to talk to other fields (for example, FAT* has an entire workshop devoted to “translation” this year), I can’t tell you the number of times I’ve talked to influential machine learning researchers who have asked, “What’s STS?” Or, if you read the literature on AI ethics education, almost all of the pedagogy is rooted in teaching “what is [insert your favorite ethical theory here] and how can you use it to justify your design choices?” It’s really hard to know what we don’t know, and I strongly believe these communities have more tools at their disposal than they think.
So, there are a few questions I’d like to explore in this space:
- What does “ethics of AI” mean to different fields (such as AI/ML, STS, philosophy, anthropology, human computer interaction, psychology, etc)? What do these different fields believe the role of ethics should (or can) be?
- What expectations do different fields have when we talk about “designing ethical AI?” What do they think is feasible?
- Can we find ways to more easily translate these definitions, tools, and expectations between fields?
- How do these concerns align with public concerns and expectations around AI? Can we translate from the communities who actively work in these areas to the public at large, and back?